Part-2: Price and Discount Optimization: Advanced AI Process
In our previous blog post, “Evidence-Based Decision Making: Revolutionizing Pricing and Discount Strategies with Causal AI,” we explored how businesses can leverage Causal AI to optimize their pricing and discount strategies. We showcased how our advanced techniques led to impressive results: a potential 6-7% increase in annual profits through optimized pricing, and an additional 1-2% increase from refined discount allocation. Today, we’re pulling back the curtain to give you an in-depth look at the advanced causal analysis process that powers these insights and drives this significant 7-9% boost in annual profits. We’ll walk you through our comprehensive approach, from data preprocessing to model evaluation, demonstrating how we uncover the true causal relationships that traditional analytics miss.
Process Overview
In our causal analysis, we navigated through an astonishing 14,641 model fits, meticulously testing 11 advanced causal AI methods. Each method requires up to 3 distinct ML models, which are selected after trying 9 different algorithms and choosing the one with the highest accuracy. This complex, nonlinear process ensures we explore every possible angle. Through a rigorous, iterative approach, we refine, retest, and validate, selecting only the top-performing models to provide the most accurate insights. This data-driven journey guarantees precision, leaving no stone unturned in uncovering the true causal factors driving the business outcomes at hand.
In the following sections, we will walk you through our comprehensive process. First, we begin with Data Cleaning to ensure data integrity. Next, we construct the Causal Graph (DAG) to map key relationships. Finally, we dive into Causal AI, where advanced machine learning models are applied to estimate causal effects with precision. Each step is meticulously designed to uncover deep insights and drive data-driven decisions.
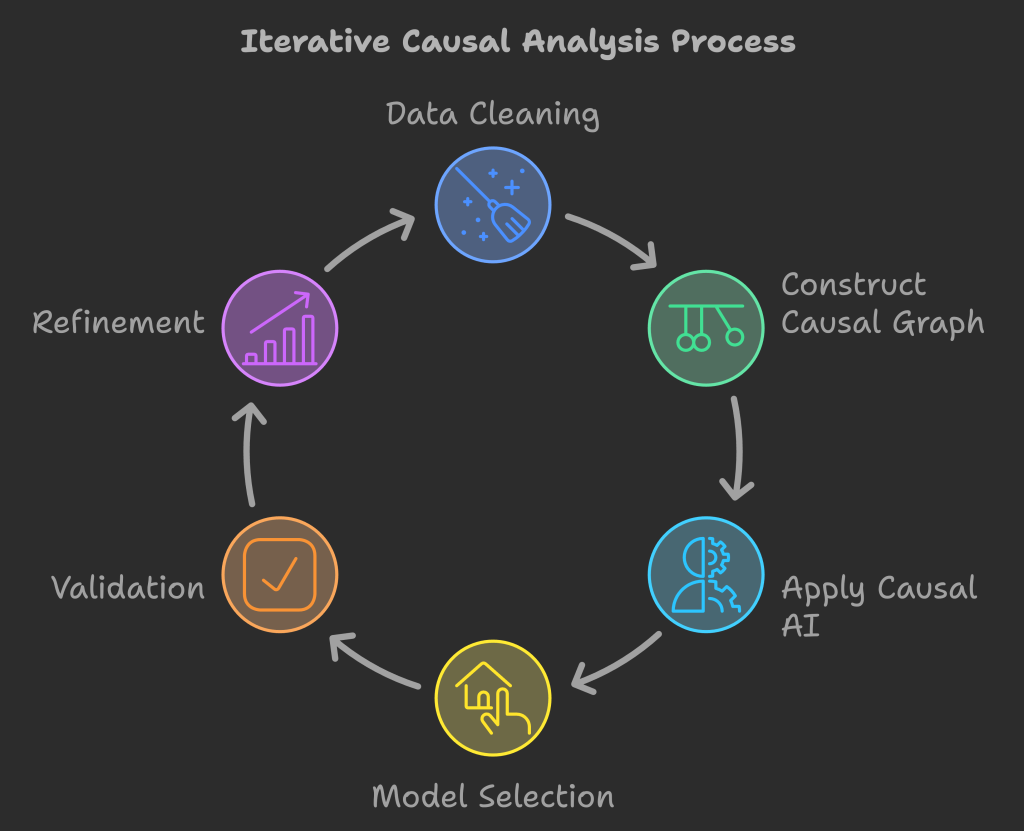
Data Cleansing
We prioritize a “data-first” approach, placing great importance on the data cleaning process. We believe in the principle “garbage in, garbage out,” meaning that even the most powerful models will not deliver accurate results if the data quality is poor. By thoroughly cleaning the data, we ensure that the models work with reliable, high-quality information, leading to more valid and trustworthy outcomes.
Based on this vision, this stage involves identifying and removing duplicate records, handling missing data through techniques like dropping or imputing values using both univariate and multivariate methods. Outliers are detected using basic visual methods such as box plots, as well as advanced techniques like Isolation Forest. Additionally, features are encoded using One Hot, Label, or Ordinal encoding, and scaling methods like Standard Scaler or MinMaxScaler are applied to standardize the data.
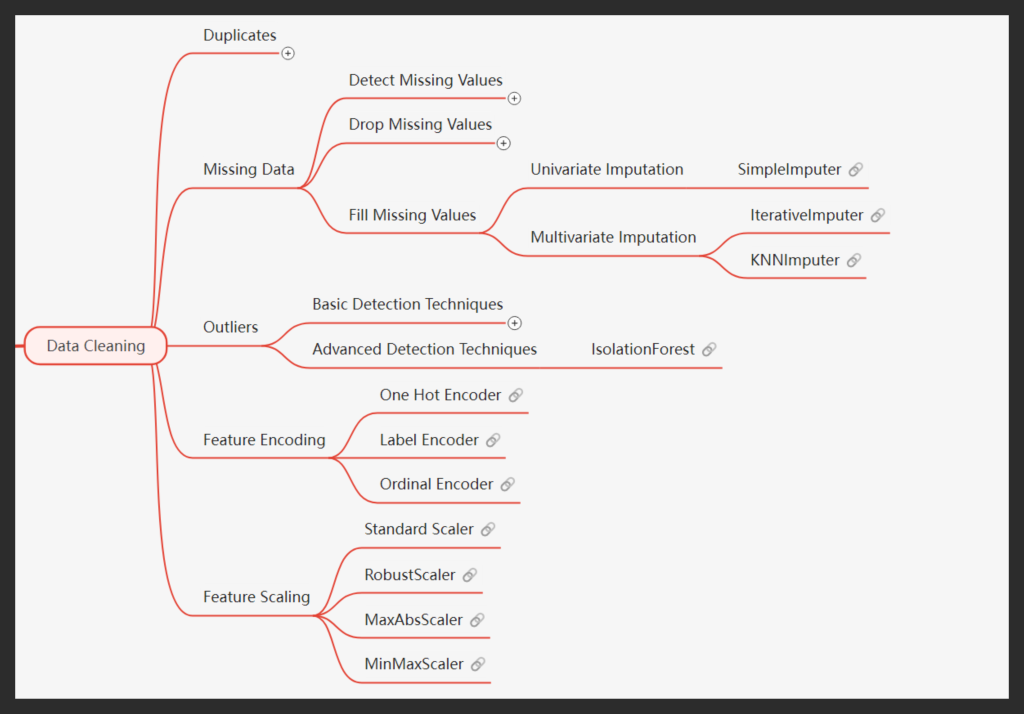
Causal AI Models
Causal Discovery and Domain Knowledge Integration: Our approach begins with automated causal discovery using the latest Algorithms, which we then complement with domain expertise in an iterative process to ensure the highest level of accuracy. We recognize that data alone cannot convey causal relationships, as causality is fundamentally a philosophical concept. Data primarily speaks the language of correlation, and without guiding this correlation toward causal understanding, we risk flawed inferences. This is why we devote considerable attention to carefully constructing causal relationships and representing them through Directed Acyclic Graphs (DAGs), which serve as the foundation for accurate causal analysis.
Causal Estimation: Once the causal structure is established, we move to the estimation phase. Here, we employ various advanced techniques, including Double Machine Learning (DML), Doubly Robust Learning (DRL), and Metalearners, each of which excels with different data types. We rigorously test all these methods to select the ones that best capture the complexities and nuances of our data. While it might be quicker to settle on just one or two models for faster results, we believe in thoroughly exploring all options to ensure the data itself determines the most suitable model for valid causal inference.
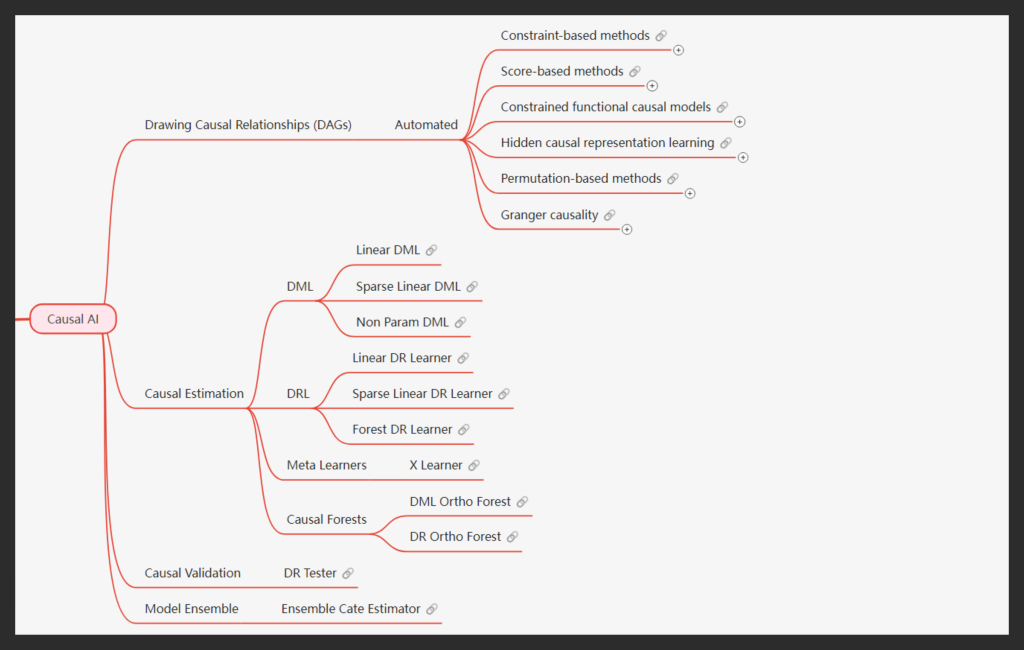
ML Models
Machine learning (ML) models form the backbone of Causal AI techniques such as Double DML, DRL, and Meta Learners. These causal models are highly flexible, allowing us to integrate any ML model of our choice. However, the selection of the ML model has a significant impact on the accuracy of causal estimates. The more accurate the input ML models, the more reliable the outputs from the Causal AI models will be.
To maximize accuracy, we don’t rely on just one algorithm for each Causal AI model. Instead, we train, validate, and test at least nine different ML algorithms with varying levels of complexity. We then select the model that delivers the highest accuracy and fine-tune it to ensure optimal performance. The range of models we work with includes everything from simple linear regressions and Support Vector Machines (SVMs) to more advanced algorithms like XGBoost and Random Forest. This rigorous process ensures we achieve the best possible results.
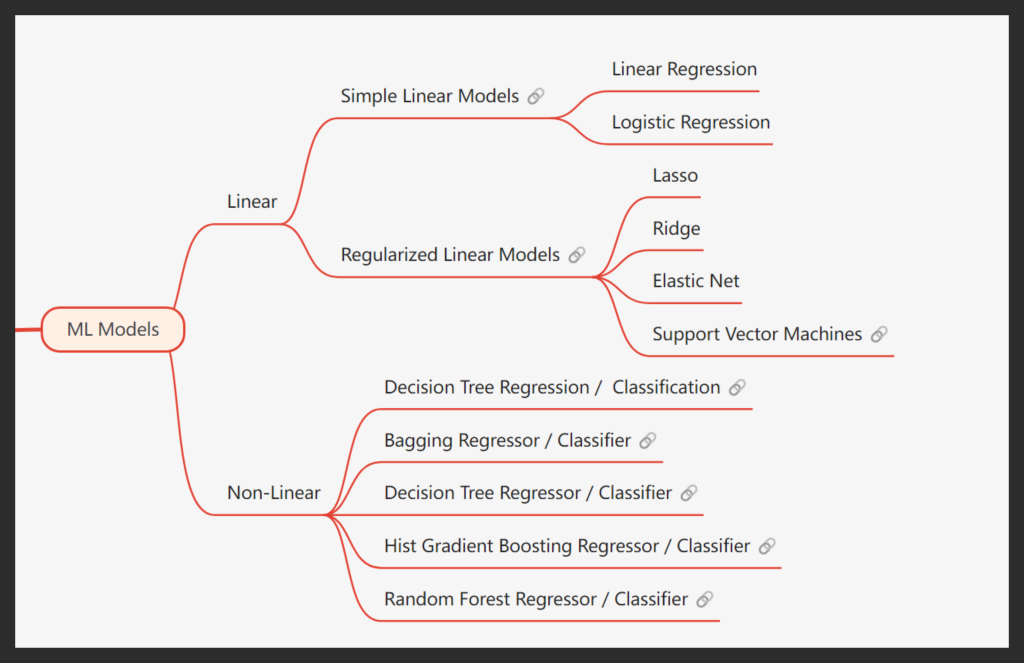
Streamline with ProfitOps AI Platform
As we have seen, the entire process of developing and validating causal AI models is inherently complex and time-consuming. It involves multiple stages, including causal discovery, the integration of domain knowledge, rigorous data cleaning, and the testing of various machine learning algorithms to identify the most accurate models. Each step requires careful attention to detail and extensive computational resources, making it challenging for many organizations to execute effectively.
However, our ProfitOps platform automates this intricate workflow, significantly streamlining the process and reducing the time and effort involved. By leveraging advanced automation, ProfitOps not only enhances efficiency but also ensures that organizations can consistently derive valuable insights from their data without getting bogged down by the complexities of the underlying methodologies. This automation allows businesses to focus on strategic decision-making, ultimately driving better outcomes and maximizing the value of their investments in causal AI.
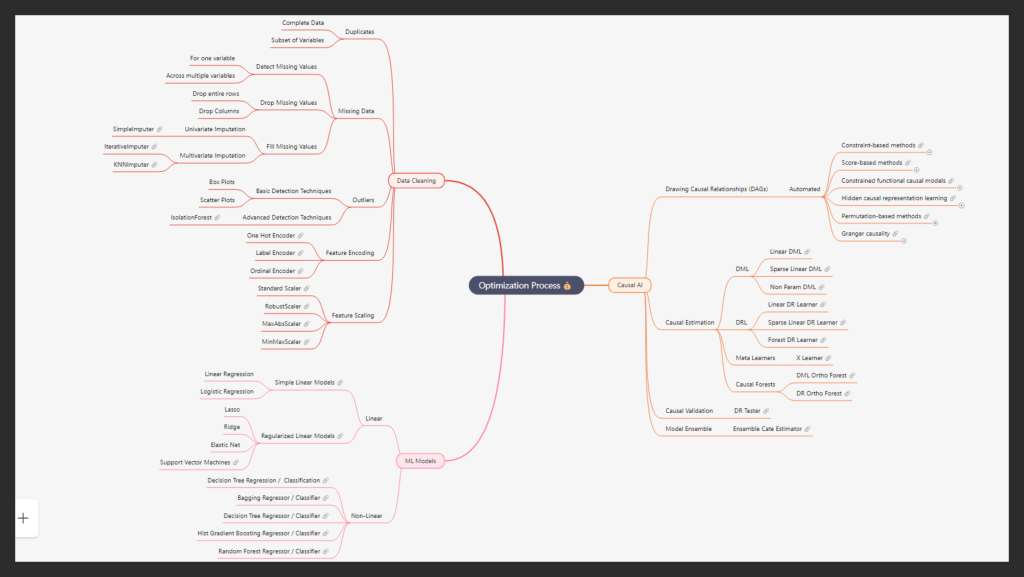
Conclusion
By leveraging our advanced causal analysis and ProfitOps automation, businesses can optimize pricing and discount strategies with precision, resulting in an estimate of 7-9% boost in annual profits. Our meticulous approach, from data cleaning to machine learning, uncovers deep insights that traditional analytics miss. With ProfitOps streamlining the process, organizations can focus on strategic decision-making, driving sustained growth and staying ahead in a competitive market.
Ready to transform your pricing and discount strategy?
Book a demo with our experts today and see how our cutting-edge solutions can take your pricing and discounting decisions to the next level!
Shravan Talupula
Founder, ProfitOps
On this page
On this page
- Revolutionizing Pricing and Discount Strategies with Causal AI
- Gut Instinct Meets Crystal Ball: Why Causal AI is the Missing Link?
- Optimizing Pricing with Double Machine Learning (DML)
- Common Discount Strategies and Their Pitfalls
- Revolutionizing Discount Strategies with Causal Estimation
- Conclusion