Part-1: Evidence-Based Decision Making: Revolutionizing Pricing and Discount Strategies with Causal AI
In the fast-evolving business landscape, making decisions based on intuition is no longer enough. Pricing and discount strategies are two of the most crucial factors that directly influence revenue and profitability, yet in many businesses today, merchandizing and demand planning teams still rely heavily on gut feelings, or the basic insights they glean from summary statistics generated in tools like Excel, Tableau, or SQL, to make critical pricing and discount decisions.
While these tools are invaluable for organizing data and generating reports, they often only scratch the surface of what’s possible. To truly optimize pricing and promotional strategies in today’s competitive marketplace, these tools should be complemented by advanced techniques like Causal Inference (Causal AI) and Operations Research (Optimization AI).
Advanced analytics doesn’t replace the insights you’re already generating—it enhances them. When used together, traditional analytics and Causal AI can unlock powerful, actionable insights that are difficult to obtain from summary statistics alone.
In this post, we’ll explore how businesses can leverage Causal AI to optimize their pricing and discount strategies, driving significant improvements in profitability and decision-making precision. In a future post, we will delve deeper into Optimization AI and explore how both Causal AI and Optimization AI can work together to unlock even greater opportunities for businesses.
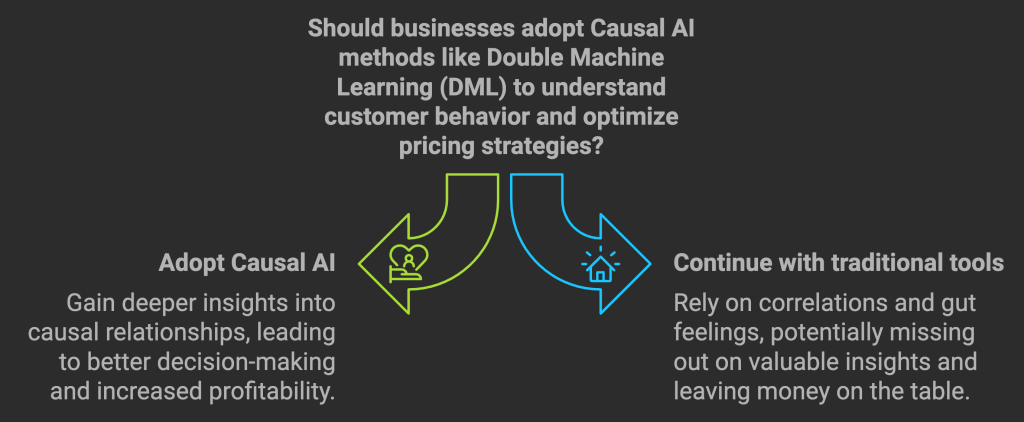
Gut Instinct Meets Crystal Ball: Why Causal AI is the Missing Link?
Let’s be clear: tools like Excel, Tableau, and SQL are incredibly useful. They allow teams to visualize trends, track performance, and make quick tactical decisions. However, when it comes to understanding the causal relationships between pricing, discounts, and customer behavior, these tools fall short. They often provide a snapshot of what has happened but don’t explain why it happened or what will happen under different scenarios.
For instance, you might see a spike in sales after a discount is applied, but was the discount the sole reason for that increase? Or were there other factors at play, such as seasonality or competitor actions? Similarly, you may notice a correlation between price changes and sales, but without understanding the underlying price elasticity, you could be leaving money on the table by setting prices too high or too low.
This is where more advanced methods within Causal AI, such as Double Machine Learning (DML), come into play. These methods allow companies to go beyond correlations and gut feelings to uncover the true, causal relationships that drive sales and profitability.
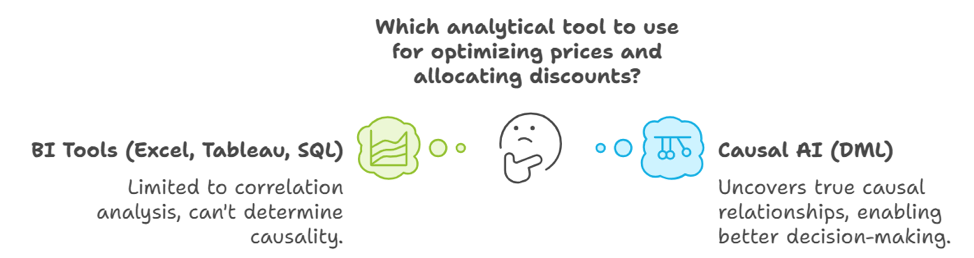
Optimize Pricing with Double ML
Businesses are adopting a holistic approach with robust optimization & cost management processes by integrating people, processes, and technology that incorporates the following:
- DML for Pricing Optimization
Double Machine Learning (DML) is a powerful tool that allows businesses to go beyond traditional methods by leveraging vast amounts of data to uncover relationships between pricing and sales. Unlike classical econometric methods, DML can handle high-dimensional data, meaning it can analyze numerous variables at once—such as customer demographics, product characteristics, and even external factors like competitor pricing or seasonality.
- Why DML Over Classical Econometric Methods?
Classical methods, like simple regressions, often assume that relationships between variables are linear and static. However, in real-world scenarios, the interactions between price, demand, and other factors are far more complex. DML not only identifies these relationships but also isolates the causal impact of price changes, providing more actionable insights.
- Benefits of DML in E-Commerce, Retail and Consumer Brands
DML is particularly powerful in e-commerce, Retail and Consumer Brands, where businesses often deal with highly varied product catalogs, customer segments, and external influences. DML can process high-dimensional data, including unstructured data like text, images, and customer reviews, to provide a more holistic view of what drives sales.
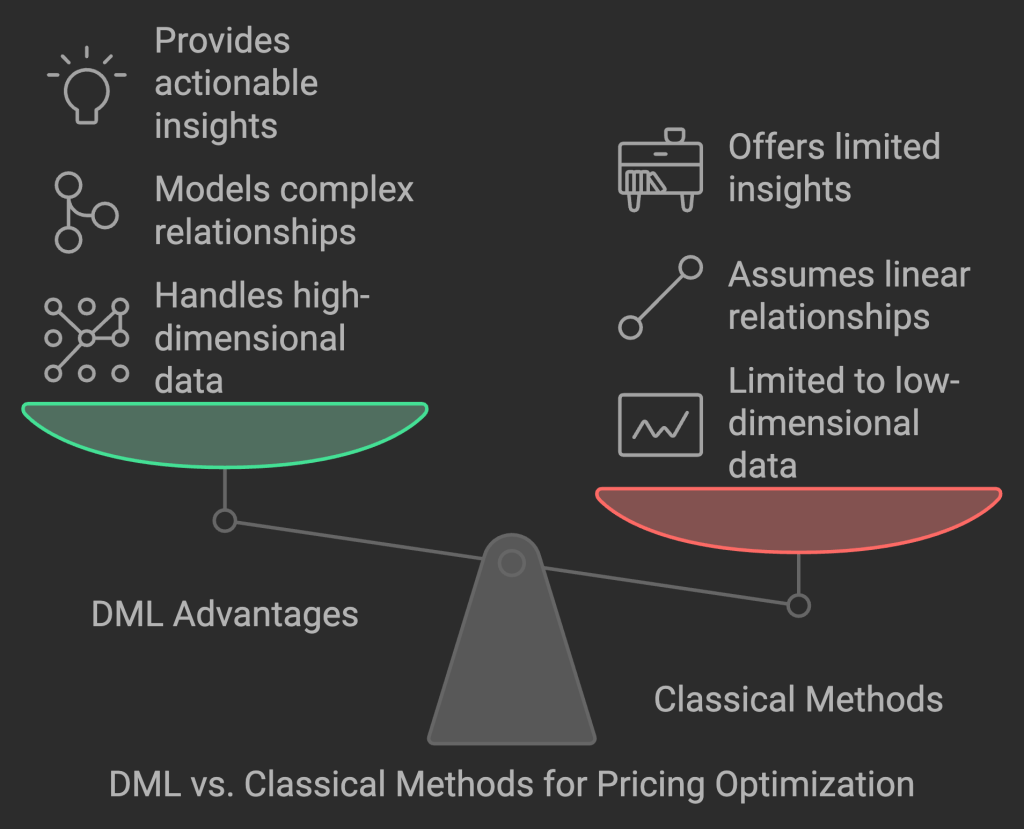
Case Study: Product-Specific Price Elasticities
One of our customers working on sales of Fitness equipment used DML to analyze the price elasticities of demand across its diverse product categories. The key findings were eye-opening:
- High price sensitivity: Products like Aerobic Steppers, Battle Ropes, Benches, and Barbells had high price elasticity, meaning that lowering prices or offering promotions on these products could significantly boost sales volume.
- Low price sensitivity: Products like Grip Training equipment, Kettlebells, Deadlifting bars, and Dip Bars were less price-sensitive, meaning that the company could increase prices without significantly reducing demand.
By adjusting prices based on these insights, the company projected a potential 6-7% increase in annual profits.
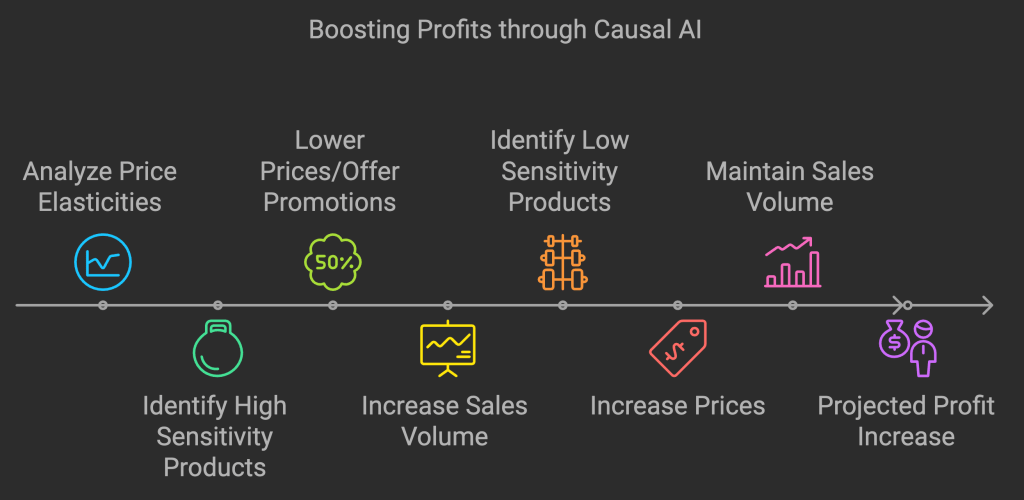
Optimize Discount with Double ML
Causal estimation allows businesses to determine the exact impact of a discount on sales and profits, taking into account a variety of external factors. DML is particularly well-suited for this task, as it can isolate the causal effect of a discount while controlling for other influences, such as customer demographics or market conditions.
Where traditional methods might show a correlation between discounts and sales, DML provides causal insights. This means businesses can confidently answer questions like, “Did the discount cause an increase in sales, or was it just a coincidence related to other factors?”
Common Discount Strategies and Their Pitfalls
- Random Discount Allocation
Randomly assigning discounts without a clear strategy can lead to wasted promotional spend. Some products may not need a discount to sell well, while others may not respond to discounts at all.
- Blanket Discounts Across All Products
Offering the same discount across an entire product line might seem like a quick win, but it often results in margin erosion. Not all products benefit equally from discounts, and applying the same rate across the board can lead to significant missed opportunities for profit.
- Discounts Only for Best-Selling Products
While it may seem logical to discount products that already sell well, this approach can backfire. Best-selling products often don’t need a discount to move off the shelf, and offering a discount here can eat into profits unnecessarily.
- Discounts Only for Underperforming Products
On the flip side, applying discounts exclusively to underperforming products can be equally ineffective. If a customer doesn’t want a product at full price, a small discount might not be enough to sway their decision.
- Problems with Measuring Discount Impact
Many businesses rely on pre-post comparisons or treated vs. untreated comparisons to measure the impact of discounts. However, these methods often fail to isolate the true effect of the discount, as they don’t account for other factors like seasonality or competitor actions.
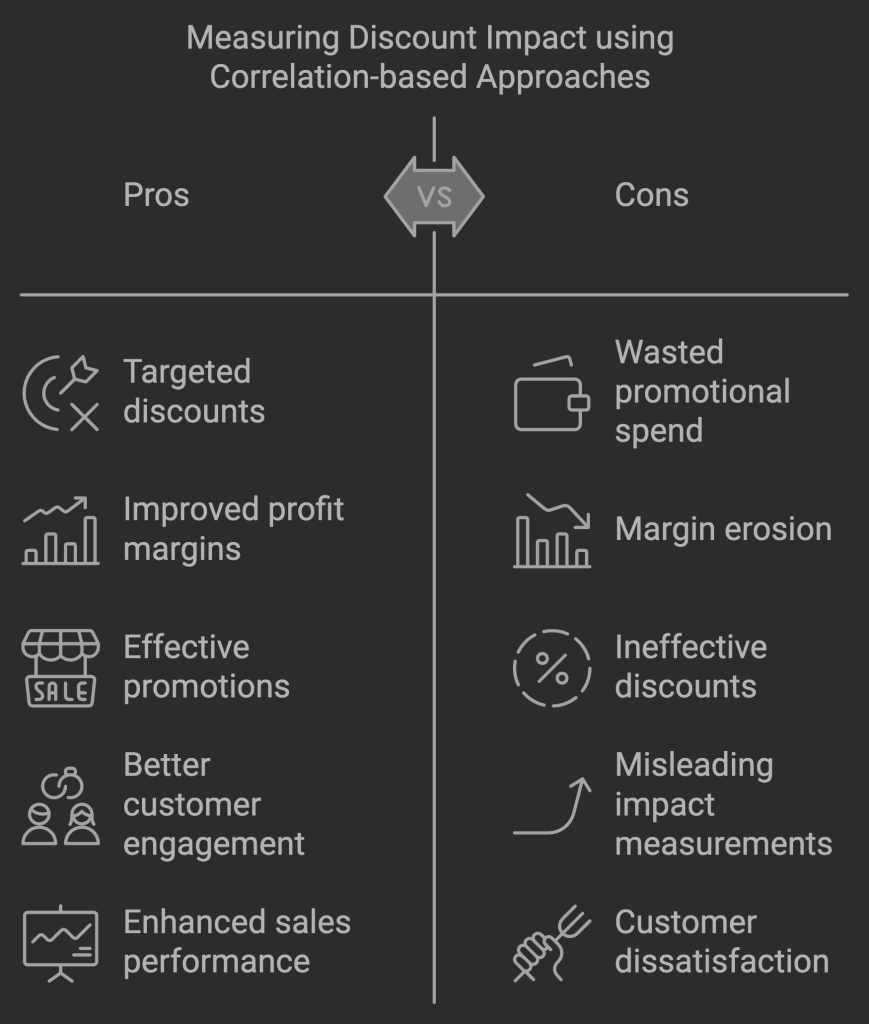
Case Study: Product-Specific Discount Allocation
One of our customers used DML to optimize its discount allocation across a wide range of fitness products. The key findings were as follows:
- Discounts on strength training equipment (benches, power racks, dumbbells) had a positive impact on sales and profitability.
- Discounts on specialized or accessory items (plyo boxes, weight plates, storage) had a negative impact, suggesting that these products were either already priced optimally or that discounts devalued these items in the eyes of customers.
- The company’s own-store products showed a stronger positive response to discounts than products sold through third-party channels like Amazon.
By reallocating discounts to the high-impact products and reducing or eliminating them on low-impact items, the company projected an annual profit increase of 1-2% —without any additional costs.
Business Results of Applying Causal AI to Pricing and Discount Optimization
By implementing Double Machine Learning (DML)-based strategies, businesses can expect more than just refined analytics—the impact on profitability can be substantial. In the case studies mentioned, the use of DML for optimizing pricing and discount strategies translated into significant bottom-line improvements.
- Discount Optimization: The optimized discount allocation led to a potential 1-2% increase in annual profits. This gain was achieved without any additional costs, simply by reallocating discounts more effectively.
- Pricing Optimization: Refining pricing strategies using DML yielded an even larger financial impact, with potential profit increases ranging from 6-7% depending on the scenario tested.
These numbers highlight how businesses can unlock hidden value in their existing data, leading to measurable improvements in profitability.
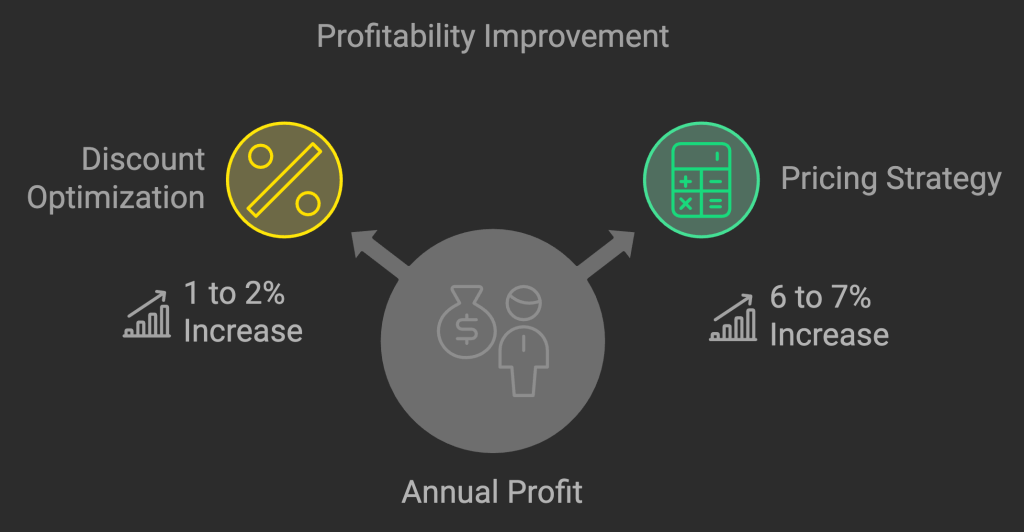
Conclusion
Advanced analytics, particularly Double Machine Learning, is revolutionizing the way businesses approach pricing and discount strategies. By moving beyond traditional methods and leveraging the power of high-dimensional data and causal estimation, companies can make more informed, data-driven decisions that lead to substantial profit increases.
For business leaders looking to stay ahead of the curve, now is the time to explore how these techniques can transform your pricing and discount strategies—unlocking new growth opportunities and boosting your bottom line.
In Part 2 of this series, we dive deeper into our advanced causal AI process, exploring how these principles are applied in practice for optimizing pricing and discount strategies.
Ready to transform your fulfillment strategy?
Discover how ProfitOps.ai can help you optimize your logistics, reduce costs, and streamline your order fulfillment processes. Book a demo with our experts today and see how our cutting-edge solutions can take your fulfillment operations to the next level!
Shravan Talupula
Founder, ProfitOps
On this page
On this page
- Revolutionizing Pricing and Discount Strategies with Causal AI
- Gut Instinct Meets Crystal Ball: Why Causal AI is the Missing Link?
- Optimizing Pricing with Double Machine Learning (DML)
- Common Discount Strategies and Their Pitfalls
- Revolutionizing Discount Strategies with Causal Estimation
- Conclusion