Digitizing Intuition with SME-Agents
Introduction: The Limits of Data-Driven Decision-Making
Over the past decade, leaders, industries, and vendors have championed the shift to data-driven decision-making. Yet the reality remains: data is NEVER complete. Even with advanced analytics and dashboards, business leaders still rely on intuition and experience to fill the gaps that data alone cannot address.
Consider this: Despite investing millions in analytics platforms, 70% of executives admit they rely on gut instinct to make major business decisions (source: HBR). Why? Because traditional business intelligence (BI) tools and digital twins—while excellent at tracking operational metrics—fail to capture the deeper reasoning, judgment, and pattern recognition that experienced professionals develop over time.
The Big Question: Can We Digitize Intuition?
Intuition—the ability to recognize patterns, anticipate outcomes, and make high-stakes decisions—has long been the secret weapon of visionary leaders. But what if we could capture this expertise, structure it, and scale it across an entire organization?
Introducing SME-Agents, a decision framework that digitizes intuition.
Unlike traditional dashboards, SME-Agents doesn’t just show data—it understands why decisions were made, remembers past outcomes, evaluates billions of alternatives, and predicts failures before they happen.
Our work introduces a hierarchical framework that integrates a structured decision template library with specialized AI agents to generate adaptive decision trajectories, thereby preparing enterprises for the era of artificial general intelligence (AGI).
Experiments in simulated retail, healthcare, and finance environments demonstrate that our framework significantly improves decision accuracy and efficiency, by complementing human expertise with an SME-Agents that mirrors and amplifies the intuitive decision-making capabilities of subject-matter experts.
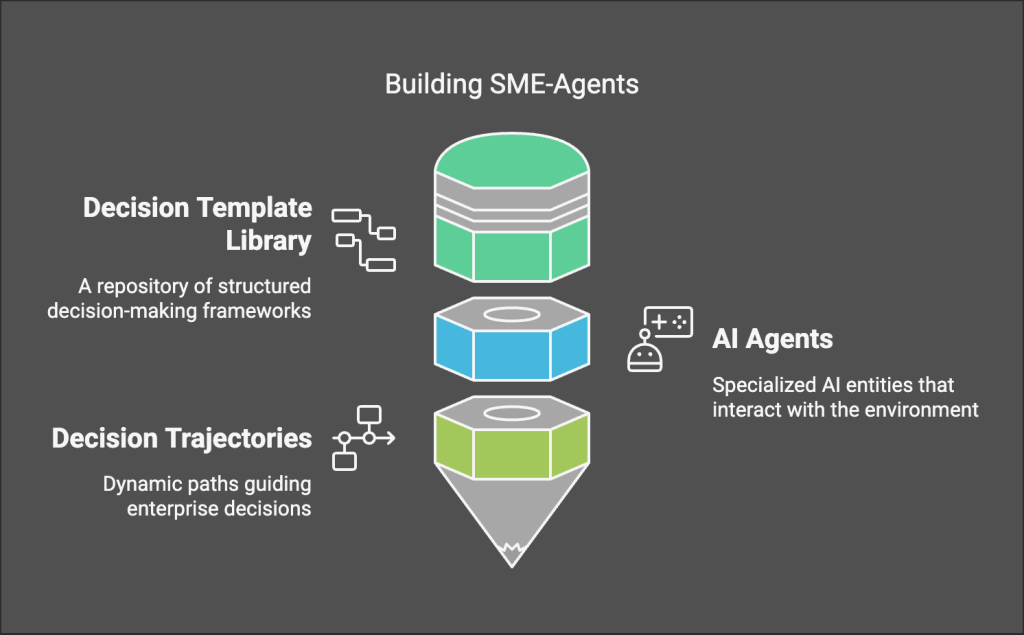
The Problem with Traditional BI & Digital Twins
Modern enterprises face decision-making challenges such as:
- Dashboard Fatigue – Too much data, too little clarify.
- Analysis Paralysis – Leaders struggle to extract actionable insights.
- Spurious Correlations – Incorrect assumptions lead to costly mistakes.
- Siloed Decision-Making – One department’s decisions impact others in unpredictable ways.
Traditional analytics platforms like Power BI and Looker are designed to track historical data—but they do not help leaders make real-time, high-stakes decisions with confidence. This is where SME-Agents changes the game.
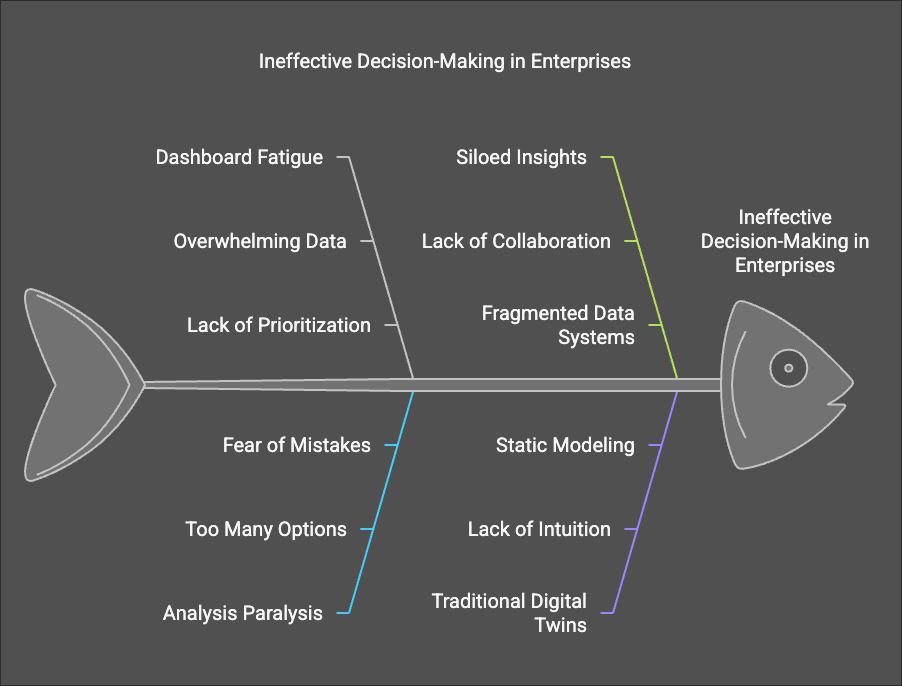
SME-Agent Framework
We propose a hierarchical decision framework that digitizes intuition by integrating multiple specialized AI agents.
We design a structured decision template library that captures expert heuristics and historical decision flows.
We develop a hierarchical reinforcement learning (HRL) process that generates optimal decision trajectories, enabling dynamic interplay between planning and execution.
We validate our approach through experiments in simulated decision-making scenarios across multiple industries.
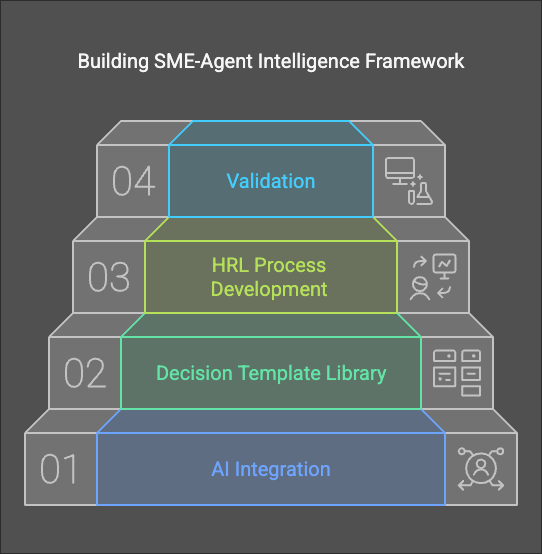
Methodology
Structured Decision Template Library
At the core of our framework is a structured library of decision templates. Each template encapsulates an expert heuristic or a proven decision-making strategy, including:
-
Template Name & Tags: For quick retrieval (e.g., “Causal Analysis in Cost Management” or “Risk Mitigation via Scenario Analysis”).
-
Description: A concise explanation of the rationale and situations in which the template applies.
-
Application Steps: A sequence of actionable steps that an expert might follow, such as identifying key performance indicators, determining interdependencies among business functions, or establishing contingency plans.
-
Historical Examples: Annotated cases demonstrating the template’s application, which help build an institutional memory.
This library enables the system to quickly recall and adapt expert knowledge to new decision contexts.
Hierarchical Reinforcement Learning for Decision Trajectory Planning:
We employ hierarchical reinforcement learning (HRL) to enable our LLM to plan an optimal decision trajectory. Given an input problem (e.g., “identify cost leaks in a retail operation”), the system first extracts key business concepts and context. Then, it retrieves a sequence of relevant decision templates from the library, forming a tentative decision trajectory. The trajectory is refined iteratively using feedback from simulated outcomes, similar to the iterative interplay seen in ReasonFlux. This process allows the system to balance exploration (considering diverse decision pathways) and exploitation (selecting the most promising strategies based on historical data).
Specialized AI Agents
To truly digitize intuition, our framework integrates four specialized AI agents, each emulating a critical aspect of expert reasoning:
-
Why Agent: Analyzes causal relationships, uncovering the underlying reasons behind observed events or cost anomalies.
-
Memory Agent: Maintains a real-time decision repository that records past decisions, actions, and their outcomes, thereby creating an institutional memory.
-
Evaluation Agent: Simulates over one billion alternative scenarios for each decision, enabling comprehensive risk–reward analysis.
-
Failure Prediction Agent: Uses predictive analytics to identify potential failure modes, allowing preemptive adjustments to the decision trajectory.
These agents work in tandem, with the HRL process orchestrating their inputs to generate a coherent and adaptive decision strategy.
Inference Scaling and Iterative Refinement
Our framework employs an inference scaling system where the retrieved decision templates are dynamically instantiated and refined. The system iteratively interacts with the decision environment (or simulation), incorporating feedback at each step. This iterative refinement mirrors human intuition, which adapts based on intermediate results and new information.
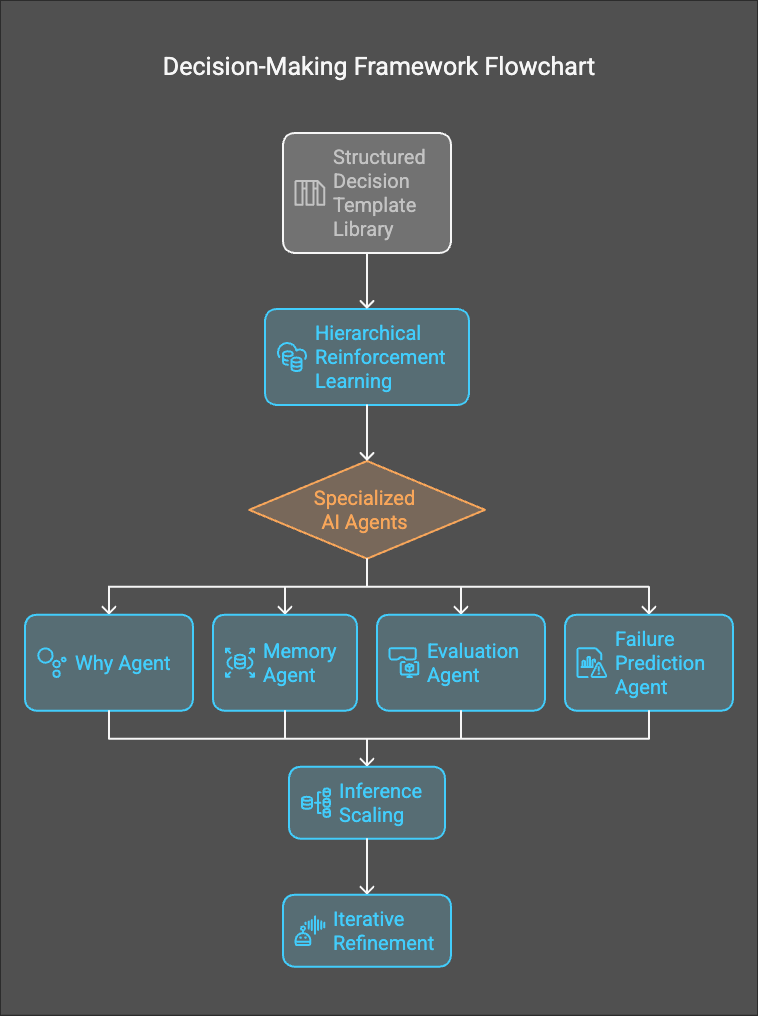
Conclusion
Our approach demonstrates that digitizing intuition through hierarchical decision templates and specialized AI agents can substantially enhance enterprise decision-making. By emulating expert reasoning and leveraging institutional memory, enterprises can reduce reliance on subject-matter experts and navigate complex, multi-dimensional challenges more effectively.
There remain open questions regarding scalability and the integration of real-time data from heterogeneous sources. Future work will explore these issues and extend the framework to incorporate adaptive learning from live operational data, further bridging the gap between human intuition and machine intelligence.
Ready to revolutionize your decision-making?
Explore how our platform can help you identify root causes, optimize logistics, and minimize operational disruptions. Schedule a demo with our experts today and discover how our cutting-edge solutions can elevate your fulfillment processes to new heights!
Shravan Talupula
Founder, ProfitOps